Causal analysis of debiasers
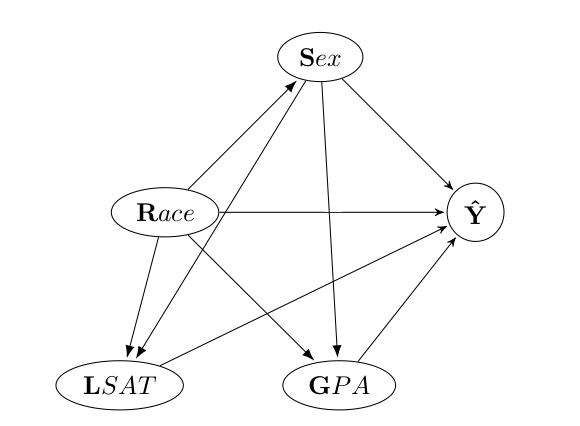
Recent research has shown, that debiasing methods often do not reliably increase fairness in practical applications while simultaneously decreasing a model’s accuracy. We study the effect of debiasing methods through a causal lens in order to develop a better understanding of factors determining whether a debiasing method works as intended. Simultaneously, a causal perspective on the phenomenon introduces the necessity to differentiate in debiasing methods, between discriminatory and non-discriminatory causal effects e.g. based on business necessity. Using this perspective on path specific effects, we study the effects of different debiasing methods on the underlying causal path specific effects (PSEs), observing empirically that reweighing reduces the direct effect of the protected attribute on the predicted label, while other PSEs are simultaneously increased. We provide an explanation for this phenomenon using an information theoretic approach. This perspective opens up the discussion for a need of incorporating causal perspectives into the development of debiasing methods in order to better capture the need for differentiating between discriminatory and non-discriminatory causal pathways.